Author: Neda Eskandari Ph.D., Paravision Machine Learning Team Lead
Note: All images in this blog post were AI-generated using DALL·E 2 and Canva’s Stable Diffusion image generator.
From celebrity deepfakes and fabricated news to generative AI art and social media filters, 2022 was truly the year deepfakes became mainstream. Since the term deepfake was born in 2017, synthetic media relying on deep learning technologies, such as generative adversarial networks, or GANs, has become more convincing and accessible at a staggering speed. One study showed volumes of deepfakes on the internet doubling every six months, a pace unlikely to slow down any time soon. Use cases of deepfakes vary from beneficial and entertaining to harmful and malicious, but what are the practical opportunities, threats, and protections of synthetic media and deepfakes?
Opportunities for deepfakes and synthetics
Perhaps the most familiar positive examples of deepfakes and synthetics come from the entertainment industry. Movie producers have long used CGI, and the industry has already started experimenting with deepfakes. With the help of deepfakes, movie producers can enable highly convincing aging or de-aging of actors without any makeup effects or even bring back late actors posthumously.
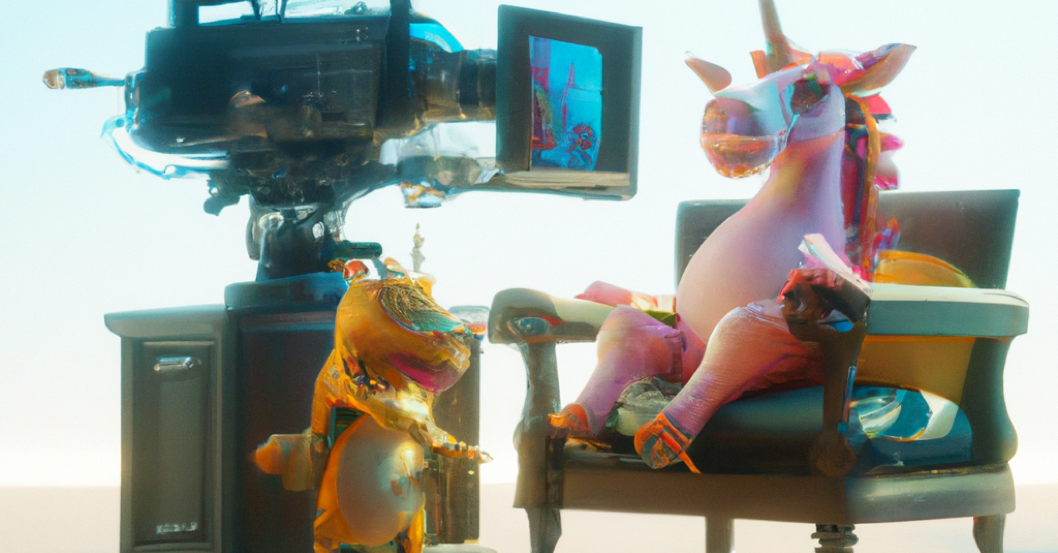
Some promising examples of synthetic media can also be found in the healthcare industry. In one example of health education, David Beckham fluently speaks nine languages in an awareness campaign for Malaria, a skill he got with the help of an expression swap model. In healthcare, scientists have suggested that deepfake audio can restore speech for those who have lost it due to a neurological illness.
In the world of technology, we’re particularly interested in the opportunities of utilizing synthetic faces as an alternative source of training data for AI algorithms. Paravision’s research team has studied whether synthetic faces could be used to expand training and benchmark datasets of face recognition models. While we aren’t yet using synthetic data in our production models, we found that with careful selection and usage, it is possible and, in select cases, can improve the accuracy of an algorithm. The finding can have significant implications in diversifying training datasets and reducing bias in AI algorithms.
Threats of deepfakes and synthetics
A powerful technology like deepfakes comes with a significant threat of malicious parties misusing the technology.
We’ve seen multiple examples from just this year of how deepfakes can threaten national security and democracy. After a deepfake of Ukrainian president Volodymyr Zelenskyy surfaced suggesting Ukrainian forces stand down during the war against Russia, government organizations globally realized the risks a well-executed deepfake of a world leader could pose. False claims have long been a part of political elections, and we’ve seen how quickly fabricated news can now spread through social media platforms. With deepfakes quickly becoming difficult for the human eye to detect, we can only imagine the impact on democracy deepfakes can have in the future in our already divided democracies.
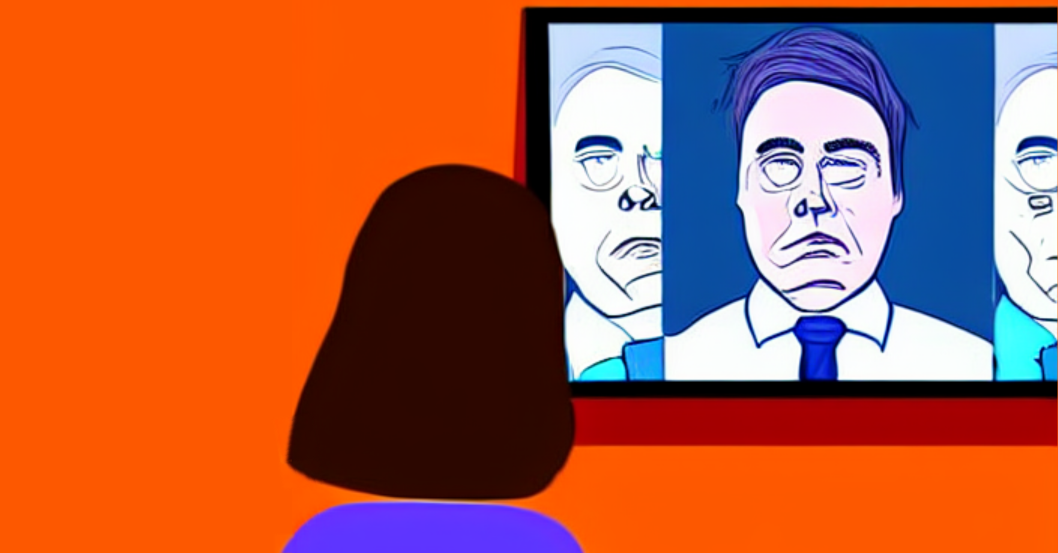
Last year, Gartner predicted that 20% of successful account takeover attacks in 2023 would be made with the help of deepfakes. In a separate study earlier this year, a Lancaster University researcher found that people trust a synthetic face more than a real person in an online setting. Earlier this summer, the FBI warned about deepfakes being used on Zoom and Teams to counterfeit job interviews with talent acquisition professionals. Combined, these studies and news give an idea of the significant financial threats deepfakes pose and the business challenges these threats can entail. Banking, security, and identity industries must pay attention to this emergent risk and integrate protections to prevent an increase in fraud and theft.
Lastly, perhaps the gravest and saddest threat of deepfakes is the privacy threat for the individuals in deepfake videos, often put into fabricated situations without consent. One study showed that 96% of deepfake videos on the internet are pornographic, disproportionately affecting women. The impact a well-executed, malicious deepfake can have on a private citizen can be devastating and destroy careers, families, and private lives. This alone should be enough reason for companies, industries, and governments to come together to develop protections against the malicious use of deepfakes.
Protections
As it gets more difficult for the human eye to detect synthetic media from real videos, many companies and governments have started building protections against deepfakes, including technology tools as well as policies toward criminalizing unconsented deepfakes.
Earlier this year, Paravision announced funding from a government agency partner to build a deepfake detector. In six months of research, the Machine Learning team has made significant progress. I recently joined The National Institute of Standards and Technology’s (NIST) International Face Performance Conference to present our research, and you can find my technical presentation here.
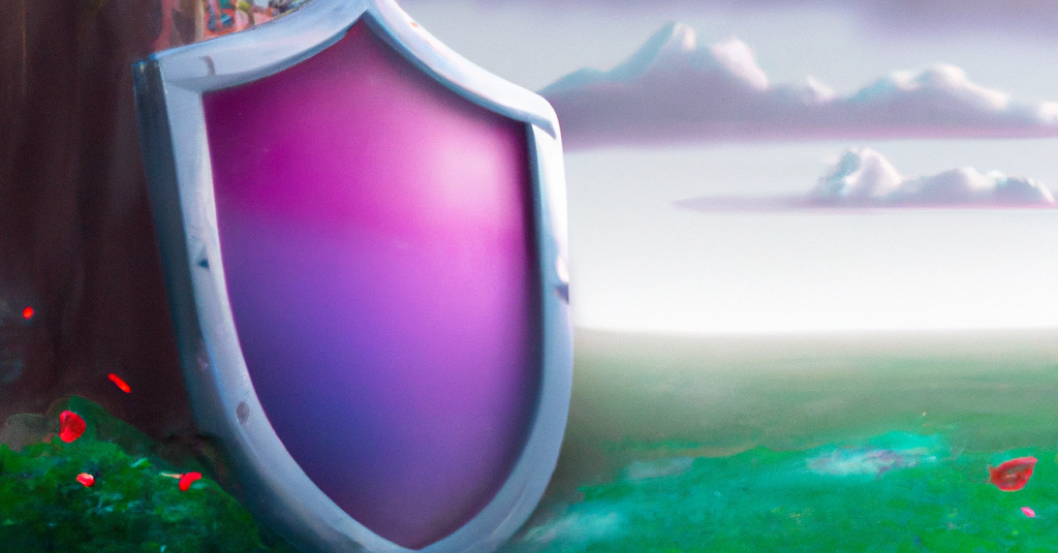
The work continues – even though progress is taking place, the lack of access to production-grade deepfake data makes it challenging for companies to build responsible, accessible deepfake detectors that would work well in real life. At the same time, as deepfake generation tools improve, deepfake detectors also need to develop at a high pace to keep up. Stay tuned and follow along as Paravision keeps making more progress on a world-class deepfake detector that can help companies across industries have protections against the threats to our security, democracy, privacy, and identity.
If you are interested in hearing more about Paravision’s deepfake detection capabilities, reach out via paravision.ai/contact.